Harnessing the Power of Agriculture Dataset for Machine Learning
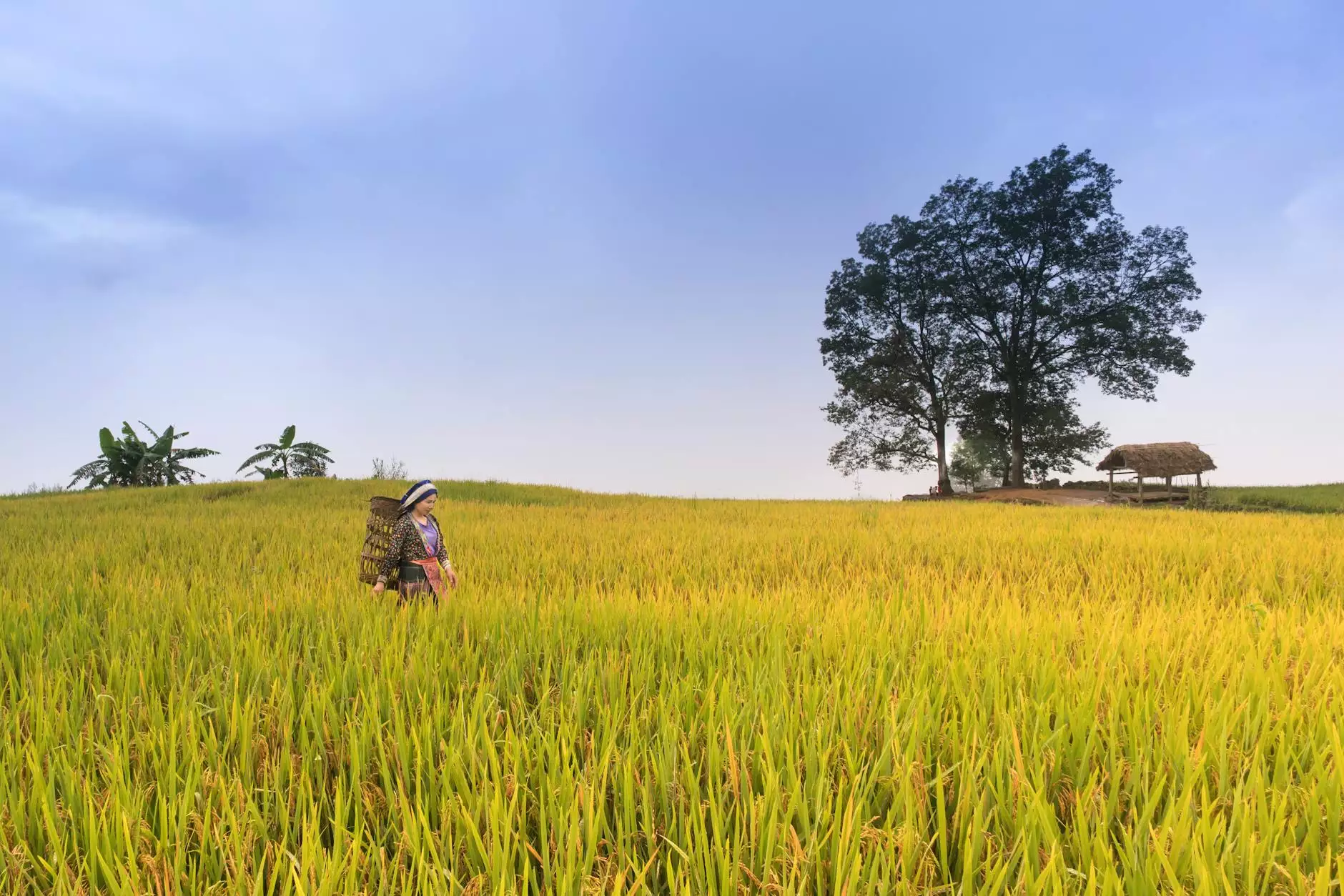
In the rapidly evolving world of technology, machine learning has emerged as a revolutionary tool, particularly in sectors where data availability and precision are crucial. One such sector is agriculture, where the application of machine learning techniques on diverse datasets is paving the way for innovative practices, enhancing productivity, and ensuring sustainability. This article delves into the significance of the agriculture dataset for machine learning, exploring its various aspects, applications, and future potential.
Understanding the Importance of Agriculture Datasets
The foundation of any successful machine learning model lies in the quality and relevance of the dataset used. In agriculture, these datasets can encompass a vast range of information, including:
- Soil health indicators: pH levels, moisture content, and nutrient composition.
- Crop growth parameters: growth rates, yield estimates, and disease prevalence.
- Weather patterns: temperature, rainfall, and humidity levels.
- Pest and disease records: infestation data and treatment outcomes.
- Market prices: trends in crop pricing and demand forecasting.
These datasets not only help in understanding current agricultural practices but also enable predictive analytics that can significantly enhance decision-making processes.
The Role of Machine Learning in Agriculture
Enhancing Crop Management
Machine learning algorithms, when applied to agriculture datasets for machine learning, allow for comprehensive analyses that can lead to improved crop management. By identifying patterns and making predictions based on historical data, farmers can optimize:
- Planting schedules: Analyzing past yield data helps in determining the best planting time.
- Resource allocation: Efficient use of water, fertilizers, and pesticides, minimizing waste and costs.
- Pest control measures: Anticipating pest outbreaks and applying treatments proactively.
Precision Agriculture
One of the most groundbreaking applications of machine learning in agriculture is in the realm of precision agriculture. By utilizing satellite imagery and sensor data analyzed through machine learning models, farmers can:
- Monitor crop health: Identifying stressed areas in fields through real-time imagery analysis.
- Optimize yields: Tailoring interventions based on localized data, thus enhancing productivity significantly.
- Reduce environmental impact: By optimizing resource use, precision agriculture minimizes chemical runoff and promotes sustainable practices.
Applications of Agriculture Datasets in Machine Learning
Yield Prediction
One of the primary applications of agriculture datasets is in yield prediction. By analyzing historical yield data along with weather and soil conditions, machine learning models can predict what yields farmers can expect from their crops. This not only helps in planning but also in financial forecasting and resource allocation.
Disease Detection
Using machine learning, farmers can utilize image recognition technology to identify crop diseases early. By training models on datasets containing images of healthy and diseased plants, these algorithms can accurately classify plant health, leading to timely interventions that can save significant portions of crops.
Automated Farming Techniques
Automation in agriculture, driven by machine learning, is changing the landscape of farming. Robots and drones equipped with machine learning capabilities can:
- Plant seeds: Using precision methods to ensure optimal planting depth and spacing.
- Monitor soil health: Continuous tracking of soil parameters to maintain optimal conditions for crop growth.
- Harvest crops: Automated systems are being developed to harvest crops at their peak ripeness.
The Future of Agriculture with Machine Learning
Looking ahead, the integration of agriculture datasets for machine learning will play a pivotal role in transforming the agricultural sector. Here are some anticipated advancements:
Improved Sustainability
As machine learning models evolve, their capacity to analyze vast datasets will enhance precision in farming. This will lead to:
- Minimized waste: Resource allocation will be more efficient, reducing excess use of fertilizers and water.
- Enhanced biodiversity: Strategies can be developed to promote crop rotation and polyculture, enhancing soil health.
- Optimized carbon footprint: The industry may see a significant reduction in greenhouse gas emissions through efficient farming practices.
Increased Access to Data
With the increasing availability of agricultural datasets, especially from governmental and research institutions, smallholder farmers will gain access to advanced technologies that were previously reserved for large-scale operations. Initiatives aimed at providing training and resources on data use for these farmers are crucial for equitable growth in the sector.
Challenges in Utilizing Agriculture Datasets
Data Quality and Accessibility
While the potential of agriculture datasets for machine learning is immense, challenges such as data quality and accessibility still exist. Poor-quality data can lead to inaccurate models and misleading insights. Ensuring that datasets are:
- Accurate: Data must be collected and processed accurately to ensure reliability.
- Current: Farming practices and environmental conditions change quickly; hence datasets must be up-to-date.
- Accessible: Efforts must be made to make data available to all farmers, especially smallholders.
Integration of Technology
Adopting new technology requires knowledge and infrastructure, which can be a barrier for some farmers. Extensive training programs aimed at teaching farmers how to utilize these technologies effectively are vital for maximizing benefits.
Conclusion
In conclusion, the impact of agriculture datasets for machine learning is profound and far-reaching. As the industry continues to harness these datasets, we can expect enhanced productivity, improved sustainability, and a more equitable future for farmers. With ongoing advancements in technology and increasing accessibility to high-quality data, the agricultural landscape is poised for a revolutionary transformation.
As we look to the future, it is crucial for stakeholders in agriculture, policymakers, and technology developers to collaborate effectively, ensuring that the potential of these datasets is realized for the betterment of the entire agricultural ecosystem.