Understanding Machine Learning Malware Detection
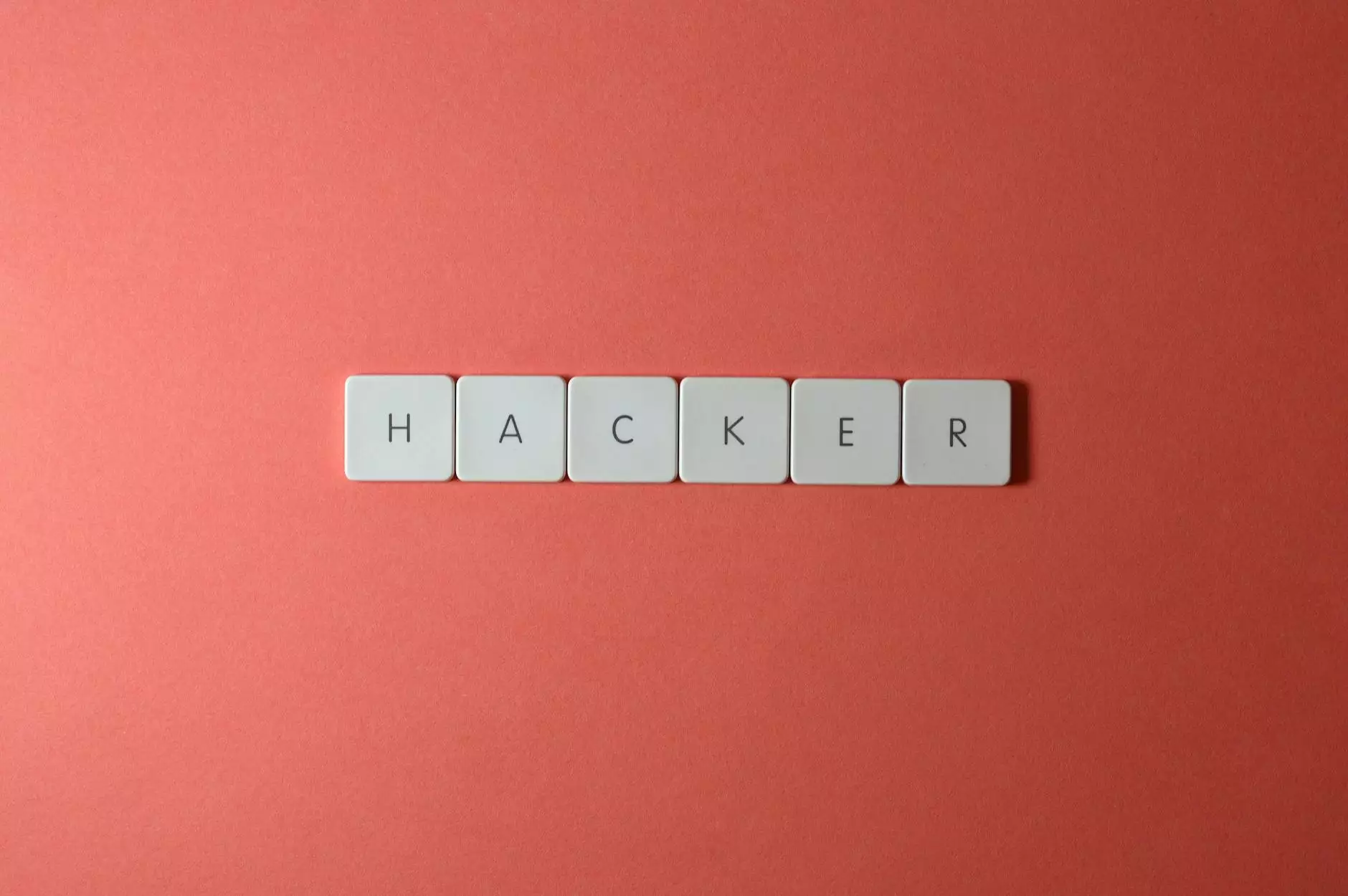
In today's digital age, the importance of cybersecurity cannot be overstated. With businesses increasingly reliant on technology, the threats posed by malware have escalated dramatically. One of the most progressive approaches to combating these ever-evolving threats is machine learning malware detection. This sophisticated methodology harnesses the power of artificial intelligence to analyze patterns and identify potential threats before they can inflict damage.
What is Malware?
Malware, short for malicious software, is a broad term that encompasses various harmful programs, including viruses, worms, trojans, ransomware, and spyware. These programs are designed to infiltrate, damage, or disable computers and networks. The consequences of malware infections can be devastating, leading to data breaches, lost revenue, and long-term damage to a business's reputation.
The Role of Machine Learning in Cybersecurity
As organizations face increasingly advanced cyber threats, traditional methods of malware detection are often insufficient. This is where machine learning malware detection comes into play. By utilizing algorithms that learn from historical data, organizations can develop models that recognize patterns indicative of malicious behavior.
How Does Machine Learning Malware Detection Work?
Machine learning involves training a model on a dataset that includes both benign and malicious software samples. This process typically consists of the following steps:
- Data Collection: Gathering a vast amount of data that includes known malware samples and normal software functioning as control data.
- Feature Extraction: Analyzing the collected data to extract relevant features that can be indicative of malware, such as file size, execution time, and system calls.
- Model Training: Using the extracted features to train a machine learning model. Various algorithms can be applied, including decision trees, support vector machines, and neural networks.
- Prediction and Classification: The trained model is then employed to classify new software in real-time, predicting whether it is benign or malicious based on learned patterns.
- Continuous Learning: Machine learning systems can adapt and improve over time by learning from new data, thus enhancing their ability to detect previously unknown malware.
Benefits of Machine Learning Malware Detection
The implementation of machine learning malware detection offers several substantial benefits:
- Proactive Threat Detection: Unlike traditional methods, which often rely on signature-based detection that can miss new threats, machine learning can identify zero-day vulnerabilities by analyzing behavioral characteristics.
- Increased Accuracy: With the ability to learn from vast datasets, machine learning models can reduce the rate of false positives and negatives, leading to more accurate threat detection.
- Scalability: As organizations grow, the potential attack surface increases. Machine learning systems can efficiently scale to accommodate more data, maintaining robust detection capabilities.
- Adaptability: The cybersecurity landscape is constantly evolving. Machine learning-powered systems can adapt to new types of malware and tactics used by cybercriminals, ensuring a level of resilience against future threats.
- Reduced Response Time: With real-time analysis and detection, organizations can respond more swiftly to threats, minimizing potential damage and loss.
Challenges Faced in Machine Learning Malware Detection
Despite its advantages, machine learning malware detection is not without challenges:
- Data Quality: The effectiveness of machine learning relies heavily on the quality of the data used for training. Inaccurate or biased data can lead to poor model performance.
- Model Complexity: Designing models that are sufficiently complex to recognize sophisticated threats can be challenging and requires expertise.
- Overfitting: If a model is too finely tuned to the training data, it may fail to generalize to new, unseen data, resulting in inaccurate predictions.
- Continuous Learning Requirements: Machine learning models require continual updates and retraining to remain effective, which necessitates ongoing resource investment.
Implementing Machine Learning Malware Detection in Your Organization
For businesses looking to bolster their cybersecurity posture with machine learning malware detection, here are key steps to consider:
1. Assess Your Current Security Infrastructure
Understanding the current state of your cybersecurity measures can help identify gaps that machine learning solutions can fill. Conducting a thorough security audit is advisable.
2. Choose the Right Machine Learning Tools
There are various machine learning platforms and tools available that offer malware detection capabilities. Consider factors such as ease of integration, scalability, cost, and the support available from vendors.
3. Train Your Team
To maximize the benefits of machine learning, ensure your team possesses the necessary skills. This may involve training existing staff or hiring new expertise in data science and cybersecurity.
4. Monitor and Update Continually
Cyber threats evolve rapidly, and maintaining an effective machine learning-based detection system requires regular monitoring and updates. This ongoing process helps refine algorithms and improves detection rates over time.
The Future of Machine Learning in Malware Detection
The landscape of cybersecurity is shifting as machine learning becomes a cornerstone of effective malware detection strategies. Emerging trends indicate a greater reliance on artificial intelligence and machine learning technologies to enhance incident response capabilities, improve threat intelligence, and analyze massive datasets with unprecedented speed and accuracy.
As organizations increasingly adopt these technologies, the integration of machine learning into malware detection systems will likely lead to more sophisticated approaches and methodologies. This reality emphasizes the need for businesses to stay ahead of the curve by investing in cutting-edge technologies and continuing to cultivate expertise in data analysis and cybersecurity.
Conclusion
In conclusion, machine learning malware detection stands at the forefront of the battle against cyber threats. By leveraging the power of artificial intelligence, businesses can effectively enhance their security measures, ensuring they can detect and respond to malware threats in real-time. As we move forward, it is imperative that companies prioritize their cybersecurity strategies, seeking innovative solutions that safeguard their most valuable assets.
Organizations like Spambrella are leading the charge in IT services and security systems, providing businesses with the tools and expertise needed to navigate the complex landscape of modern cybersecurity. Embracing machine learning for malware detection not only fortifies your defenses but also positions your business for success in an increasingly digital world.